- 8 marzo, 2023
- Posted by: Oscar Becerril
- Categoría: Economics
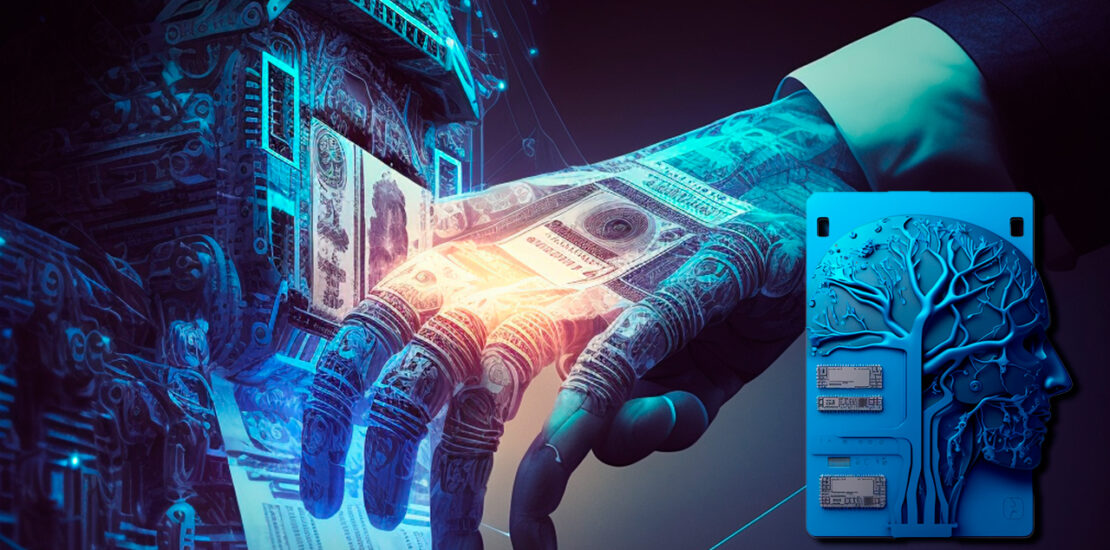
*Artificial intelligence was used in the research, image and drafting of this article
Credit is one of the most important activities in the banking industry. It has the most direct impact on its operating results, is a source of revenue and financial margin, and has a strong impact on the economic activity of people, business and companies, with a natural consequence in a nation’s GDP.
The credit process has become one key activity in terms of the assertiveness that banks and financial companies must have in order to achieve adequate and proper credit decisions. This must consider the best way to meet challenges, such as speed and effectiveness of review and approval of credit applications.
The latter becomes important when we take into consideration certain competitive factors, such as to grant credit with the opportunity that the market demands. This requires to have processes of analysis, authorization, execution and delivery of resources in a way that can assure that these processes are effective and fast enough to fit the needs of borrowers.
Historically, credit analysis has been one of the most important and challenging activities in the banking industry, considering the complexity that credit decisions can have, specially for the assessment and evaluation of credit risks, which become more complicated as businesses or project investments grow.
Credit process refers to the steps that a lender or financial institution follows to approve or decline a loan application. The credit process involves a series of stages, each of which is designed to assess the borrower’s creditworthiness and determine the risks associated with lending to them. Credit risk is also associated to the probability of default in the repayment of a loan.
The credit process typically begins with the borrower submitting an application, which includes information about their financial situation, employment status, income flows, and other relevant details. The lender then conducts a credit analysis, which may include a review of the borrower’s credit report, income and expenses, debt-to-income ratio, collateral, and other factors.
Based on this analysis, the lender will make a decision about whether to approve the loan, the loan amount, and the interest rate. The credit process may also involve ongoing monitoring of the borrower’s creditworthiness and repayment history to ensure that the loan remains a good risk for the lender. Integral management credit risk should have an impact on the price, in terms of the rate and commissions to be applied to the loan.
Credit risk refers to the risk that a borrower may default on their financial obligations, such as failing to make payments on a loan or credit card. It is the risk that a lender or financial institution takes on when lending money or extending credit to an individual, company, or organization.
Managing credit risk is essential for lenders and financial institutions to maintain a healthy balance sheet and minimize losses. Lenders use various tools and techniques to manage credit risk, including credit scoring models, underwriting standards, loan covenants, collateral requirements, and ongoing monitoring of the borrower’s creditworthiness.
Effective credit risk management involves finding a balance between approving a certain number of properly structured and secured loans to generate income and minimizing the risk of default. By managing credit risk effectively, lenders can minimize losses and maintain their financial stability over the long term.
Artificial intelligence (AI) is increasingly being used in the credit process to improve the accuracy, efficiency, and speed of lending decisions. AI can help lenders analyze large amounts of data quickly and accurately, enabling them to make more informed borrowing choices. Over time, this typically generates a better and more robust credit portfolio, which also has a positive impact on credit conditions available to all customers.
One application of AI in the credit process is credit scoring. Credit scoring models use statistical analysis to assess a borrower’s creditworthiness based on factors such as their credit history, income, and employment status. AI can help lenders develop more accurate credit scoring models by analyzing a wider range of data and identifying patterns and trends that human analysts may miss.
AI can also help lenders automate the loan approval process by analyzing loan applications and making decisions based on predefined criteria using data base in parametrical models based on previous credit experience and a diverse set of factors. This can reduce the time and cost of loan processing and improve the overall customer experience. These new trends are substantially changing the way financial institutions operate credit applications, and also the integration and training of credit teams all over the world.
Another area where AI is being used in the credit process is fraud detection. AI algorithms can analyze transactions and identify patterns of behavior that could be indicative of fraudulent activity. This can help lenders detect and prevent fraud, reducing their losses and improving the security of their lending operations. It has been observed that AI can detect frauds or abnormal activities that traditional methods cannot detect normally.
However, it is important to note that AI is not a magical solution to all problems in the credit process. The use of AI in credit decision-making raises important ethical and regulatory issues, including concerns about algorithmic bias, data privacy, and transparency. Therefore, it is crucial to ensure that AI is used responsibly and in compliance with relevant laws and regulations.
Credit plays a crucial role in the global economy as it enables individuals and businesses to access capital and invest in projects that drive economic growth. Credit provides a means of financing for various purposes, such as starting or expanding a business, buying a home, or investing in infrastructure projects.
A well-functioning credit market facilitates investment, innovation, and economic growth. It enables businesses to expand and create jobs, which in turn leads to higher incomes and increased consumer spending. Additionally, credit helps individuals and households smooth their consumption over time by enabling them to borrow to finance large purchases or unexpected expenses.
Moreover, credit promotes international trade by providing financing for imports and exports. Exporters can extend credit to their buyers, while importers can obtain credit to finance their purchases. This facilitates cross-border trade and helps to support global growth.
However, it is important to note that credit can also contribute to financial instability if not managed properly. Excessive borrowing and lending can lead to financial bubbles and crises, which can have severe consequences for consumers.
It is important to understand that AI should actually be considered as a technological tool that will be critical in credit processes such as analysis and authorization, specially for consumer credit such as credit cards. However, in the case of credits for SMEs, AI is a support tool that provides an objective analysis that translates into scoring, likelihood of default, projected cash flow and another quantitative indicators that will help to achieve better credit decisions. But the final decision still has to be in the hands of a team of experts.
It is unquestionable that AI is changing substantially the way we operate, analyze, authorize and grant credit. The use of technology should lead us to reduce credit risk and therefore the size of non-performing portfolios. This will result in having stronger and better capitalized financial institutions and even more in reducing the size and amount of reserves, with the expected outcome of having a more healthy and robust banking industry. This will eventually translate into credit in better conditions, including lower interest rates for customers.